Machine learning's potential to assist in climate change mitigation and adaptation is vast, but as with any developing technologies, so are the challenges. In this episode, we talk with journalist David Silverberg and
How AI can help fight climate change
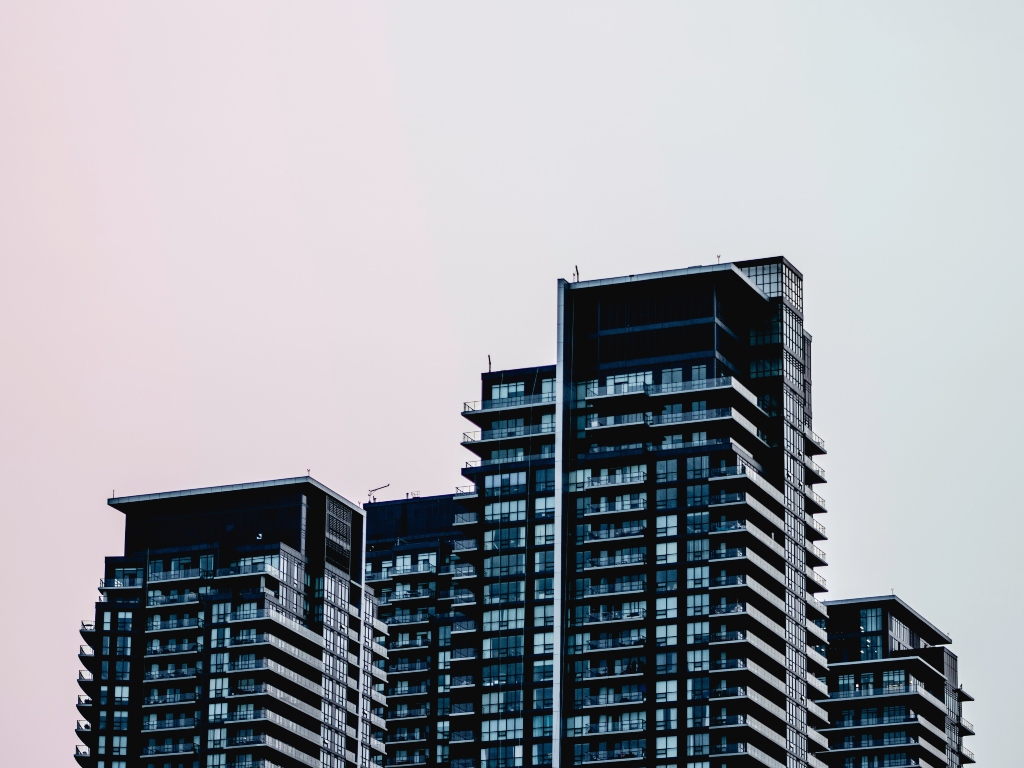
By David Silverberg
When you think of AI, images of quick-thinking chatbots and Amazon recommendations may come to mind, but there’s another key area where this innovation is finding a home: researchers and academics are harnessing machine-learning (ML) to better fight climate change.
One of the most impactful papers on the topic was published in late 2019, bringing together some of the most prominent ML experts to collaborate on a report that aims to “provide an overview of where machine learning can be applied with high impact in the fight against climate change, through either effective engineering or innovative research,” according to the introduction to “Tackling Climate Change with Machine Learning.”
The authors have noted that the paper is helpful not just for AI practitioners, but can inform a range of people who need to take part in climate change work, such as entrepreneurs, investors, and business and government leaders.
As promising as ML can be in advancing the fields of climate modeling, managing electrical systems, and optimizing solar and wind power sources, this burgeoning tech also has faces limits in how it crunches data and generates applicable solutions.
Divided by topics such as Transportation and Buildings & Cities, the paper looks at what’s occurring now in ML’s opportunity to mitigate climate change and how those examples can be expanded beyond their borders. It looks at where manual systems fail us, too.
“Turning down a thermostat by hand doesn’t lead to the most efficient energy use,” said David Rolnick, a University of Pennsylvania postdoctoral fellow and one of the authors. “In heating and cooling systems, machine learning can control the temperature in a building much more efficiently than a human can by understanding the power required to control the system.”
More accurate climate predictions is a hopeful theme in the paper, due to how often researchers have come across the challenge of limited datasets in accurate climate models. “No matter how many weather stations we construct, how many field campaigns we run, or how many satellites we deploy, the Earth will generate at most one year of new climate data per year,” the paper stated.
But an under-recognized segment of climate modeling could take advantage of ML: clouds. Priya Donti, a Carnegie Mellon PhD student and another author of the paper, referred to Columbia Engineering research that found ways to predict many of the cloud heating, moistening, and other features that are essential to climate simulation. After all, bright clouds block sunlight and cool the Earth, while darker clouds capture outgoing heat and keep the Earth warm. “In this case, and many others, machine learning can predict what’s happening by using historical data, and then try to glean insights form that to understand the future,” she said.
Donti’s contribution looked at more efficient electrical systems, which continues to be a key culprit in accelerating climate change. “Where machine learning can plug in here is to help forecast electrical supply and demand and improving how the grid is scheduled to incorporate low-carbon sources and accelerate solar fuels and liquid fuels, such as nuclear fusion,” added Donti.
Also critical is how ML can be a key player in what is called “predictive maintenance,” which means forecasting when a component of a structure may be in need of repair. Those kinds of predictions could potentially stop methane gas leaks before they happen, Donti said.
Moving ML from the lab to the real world is happening quickly, as Lynn Kaack described it. Also a contributor and editor of the paper, and postdoctoral researcher at ETH Zürich, Kaack said in an interview. “Academic research in these areas is increasing and is starting to be deployed across society. For example, in the electricity sector, UK's National Grid ESO is forecasting renewable generation based on machine learning.”
But this isn’t news to Claire Monteleoni, an associate professor in the computer science department at the University of Colorado Boulder, who helped launch the field of climate informatics. She gave a video tutorial at the Conference on Neural Information Processing Systems in 2014 where she highlighted the success and challenges facing machine learning in how it can help combat climate change.
She said, "Part of the role of AI has been to shed light on, or improve performances of existing climate models or existing results in the climate literature."
Monteleoni has seen the governmental buy-in from agencies such as NASA and the NOAA, the latter taking a very active position in hiring machine-learning researchers to advise on various projects. Private firms such as Microsoft and Google are also "stepping up to fund research in the area of AI and machine learning for climate change," Monteleoni said, citing the example of Google's flood forecasting pilot project set up in India's Patna region.
Google has been one of the leaders in this space for several years, which ML experts noticed when firm announced in 2016 that its DeepMind machine-learning system “managed to reduce the amount of energy we use for cooling by up to 40 percent… The implications are significant for Google’s data centres, given its potential to greatly improve energy efficiency and reduce emissions overall. This will also help other companies who run on Google’s cloud to improve their own energy efficiency.”
Often, giant corporations will fund initiatives bridging ML and climate change challenges. Using AI tech made possible with a Microsoft grant, Seattle-based conservation organization Long Live the Kings has compiled large datasets to analyze the conditions surrounding salmon-population depletion.
It’s not enough to collaborate on an 826-citation paper outlining what’s been successful and what’s needed next in fighting climate with ML. Donti said, “Governments, businesses, investors, and other institutions will need to take rapid and meaningful actions for society to make progress on climate change. While machine learning can provide tools to help with this transition, it fundamentally cannot take these actions or make the corresponding tough decisions for us.”
Also, simply put, machine learning technology may not be sophisticated enough to tackle the many challenges climate change throws at us. Donti said, “There are many mature ML algorithms used for forecasting, but, for example, in the energy sector, forecasts of solar and wind power need to be better integrated into the ways that electricity system operators and other relevant players make decisions.”
The report’s authors also suggested that limited datasets, whether in climate modeling or materials science, can hurt ML’s chances at accurately constructing algorithms to best predict what will happen next.
What also has to be considered is what researchers in the paper identify as Jevon’s Paradox: the concept refers to a situation where increased efficiency still results in higher overall demand. Looking at autonomous vehicles, they may cause people to drive far more, so that overall GHG emissions could increase even if each ride is more efficient.
How machine learning operates could actually hurt the environment more than help it. A recent paper by University of Massachusetts researchers found that AI models that use neural architecture search emit the carbon dioxide equivalent of nearly five times the lifetime emissions of an average American car.
Rolnick said confronting the many facets of fighting climate change with ML doesn’t just rest with paper’s publication. He said the research spawned a broader initiative called Climate Change AI consisting of an organizing team of 12 members and 12 advisors whose goal is “facilitate meaningful work at the intersection of AI and climate and bring home various stakeholders and decision makers on various fronts, whether from academia, industry or specific communities.”